Handle with Care
Predictive modeling has transformative potential in diagnosing medical conditions—but its complexities provide a tough test for scientists
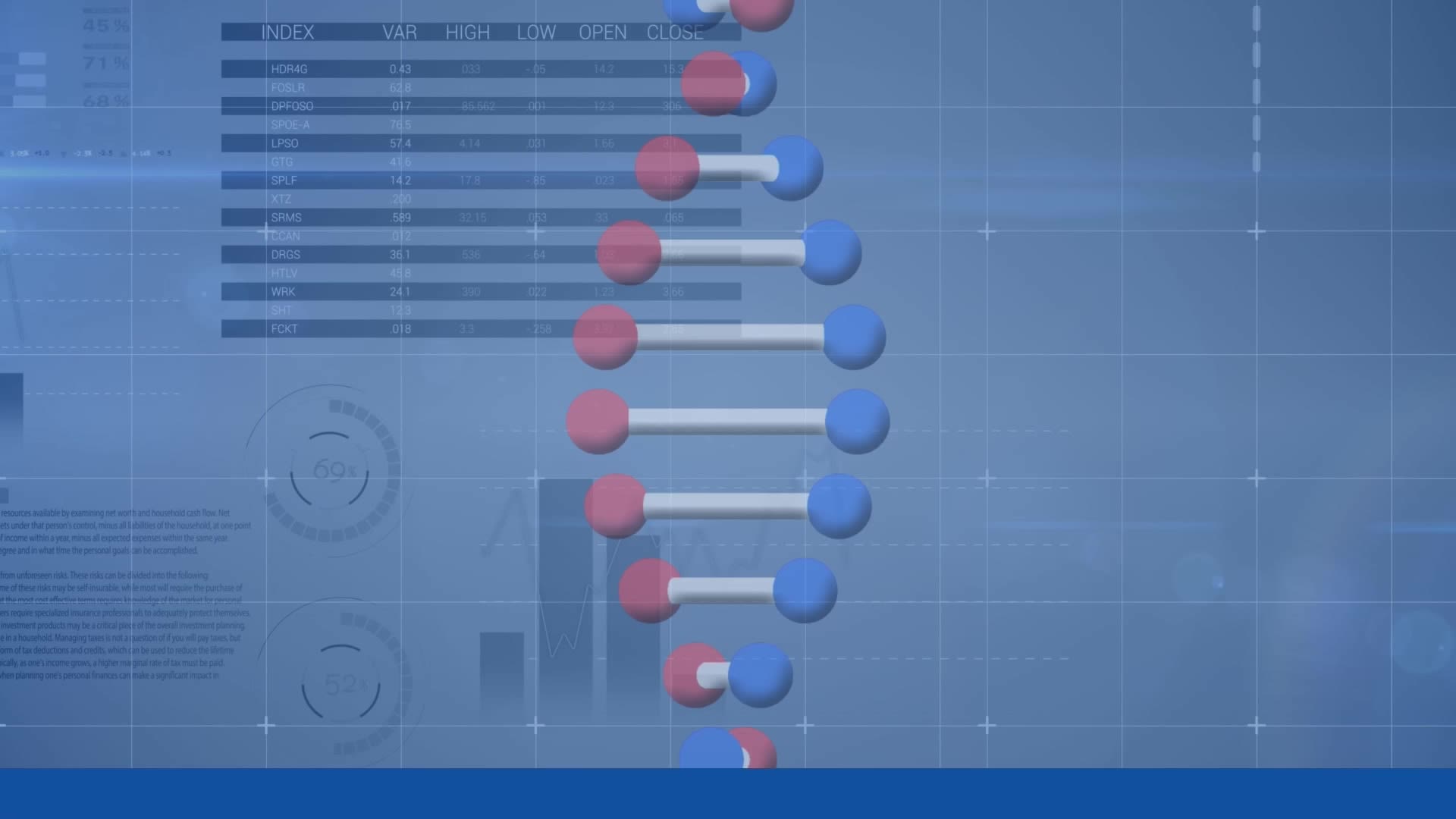
Imagine a future where potentially fatal diseases like leukemia can be predicted before any cancerous cells are present in the body. Thanks to artificial intelligence, that reality is closer than it may seem. AI has the potential to revolutionize cancer care, from early detection and diagnosis to treatment decisions. If developed and used responsibly, these systems could go a long way in improving health care delivery and patient outcomes, say Rutgers–Camden experts.
Deep learning is a type of artificial intelligence that attempts to simulate the behavior of the human brain, allowing it to “learn” from large sets of data, said Iman Dehzangi, an assistant professor of computer science with the Rutgers University in Camden College of Arts and Sciences and a leading expert in applications of AI in health care. As a scholar of bioinformatics and computational biology, he develops machine learning tools to address challenging problems in biology, including cancer detection and analysis. To do so, he trains models to analyze historical data and identify patterns that can be used to predict future outcomes.

Iman Dehzangi, assistant professor of computer science in the Rutgers University in Camden College of Arts and Sciences, and a leading expert in applications of AI in healthcare
Iman Dehzangi, assistant professor of computer science in the Rutgers University in Camden College of Arts and Sciences, and a leading expert in applications of AI in healthcare
“To create a coherent system, provide generalized outcomes, and represent the whole population, we try to collect as much data as possible. The more data, the better.”

“To create a coherent system, provide generalized outcomes, and represent the whole population, we try to collect as much data as possible,” he said. “The more data, the better.”
The problem is that the algorithms are often built on data sets that reflect inequities that have long plagued U.S. health care. In medicine, racial and ethnic minorities have long faced barriers to receiving care. Because wealthy, predominantly white individuals tend to make more use of health care, algorithms learn to flag them for extra medical attention, Dehzangi said. These biases can become immortalized in data, and deployed at scale in sensitive, high-stakes ways.
“When you are trying to build a model, it normally skews toward the majority class,” Dehzangi said. “Data can come from different sources across economic groups and ethnicities, but it likely includes some bias towards the majority group. If I have 900 samples from one ethnic group and 100 samples from all the rest, the dominant pattern represents the 900. Even if I correctly predict those 900 and incorrectly predict the rest, the model would be considered 90 percent accurate.”
These disparities are particularly pronounced in the case of breast cancer, said Bonnie Jerome-D’Emilia, an associate professor in the School of Nursing–Camden. In a paper published in the American Journal of Clinical Oncology, Jerome-D’Emilia and her co-authors wrote, “Racial and ethnic minority women continue to be diagnosed with breast cancer at a later stage and with greater tumor size and higher-grade tumors, important predictors of cancer mortality.” Reasons for these disparities are complex, but they stem from minorities’ lagging access to high-quality care.

Bonnie Jerome-D’Emilia, an associate professor in the School of Nursing–Camden
Bonnie Jerome-D’Emilia, an associate professor in the School of Nursing–Camden
“Issues like health insurance, transportation, and child care can be major barriers to seeking preventative care,” Jerome D’Emilia said. “In minority populations, we also see a distrust of health care providers in general. These individuals are less likely to have primary care providers who remind them to get mammograms and other routine screenings. All of these factors and more contribute to these groups being severely underrepresented in health care data."
While social, economic, and behavioral factors are important, Dehzangi said, evidence also suggests that demographic factors can influence the biological and molecular mechanisms of cancer. For example, a recent studyfound that eight genes responsible for DNA repair are expressed differently in tumors from Black women than tumors from white women. These molecular differences correspond with changes in how quickly breast-cancer cells can grow and have critical implications for the course of treatment.
Findings like this illustrate the need for more inclusive data sets that look at the complex interplay of biological, genetic, and lifestyle factors in each patient, Dehzangi said. The results generated by AI can be used to identify risk factors for breast cancer, make a diagnosis, and develop a treatment plan. In the context of breast cancer—the fifth leading cause of death worldwide—even the smallest missteps can have life-or-death consequences.
In a recent article published in the Journal of Biomedical Informatics, “A Review on Deep Learning Approaches in Healthcare Systems,” Dehzangi called on developers to favor “explainable AI” systems that share the reasoning behind their diagnoses, allowing stakeholders to question the underlying decision-making processes.
Dehzangi also stressed the importance of evaluating a model’s performance on more than just accuracy alone. While intuitive and easy to measure, accuracy—which essentially is the number of correct predictions divided by the total number of samples—tends to mask imbalance. Other metrics, like sensitivity and specificity, can give a fuller picture.
“Say the model is trained to interpret mammogram images,” Dehzangi said. “If most of our samples are collected from middle-age, white, Caucasian women, it’s likely that all those images would help us identify that specific pattern much more effectively. Among different ethnicities, there are different indicators of cancer. In this case, the class with a higher occurrence may be correctly predicted, leading to a high accuracy score, while the minority class is being misclassified. This gives the wrong impression that the model is performing well when it is not.”
The more we know about a model, the more we can question its inner workings and anticipate its limitations, Dehzangi said. As researchers continue to navigate this path, transparency around the sourcing, composition, and interpretation of data is key to developing AI systems that are not only intelligent, but fair and trustworthy.
“When predictive health care tools first began to emerge, there was a lot of emphasis on accuracy,” Dehzangi said. “Now, we’re seeking to answer questions like, Why are we accurate? How we are accurate? How do we explain our model? If we cannot answer these questions, how can we be sure we are serving the right purpose?”


Design: Karaamat Abdullah